Journal of Credit Risk
ISSN:
1744-6619 (print)
1755-9723 (online)
Editor-in-chief: Linda Allen and Jens Hilscher
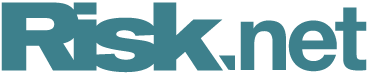
Instabilities in Cox proportional hazards models in credit risk
Need to know
- Cox Proportional Hazards models, when used to analyze data typical of loan performance, exhibit significant instabilities.
- The Partial Likelihood estimator of Cox PH models hides the potential multicollinearity between explanatory covariates and the hazard function.
- The source of the Cox PH multicollinearity problems is the same as the model specification error in Age-Period-Cohort models and will be present in any model that analyzes data from loan performance.
- The various algorithms employed for credit risk modeling generate “unique” solutions because of embedded assumptions, as with the Cox PH partial likelihood estimator.
Abstract
When the underlying system or process being observed is based upon observations versus age, vintage (origination time) and calendar time, Cox proportional hazards (PH) models can exhibit instabilities because of embedded assumptions. The literature on age–period–cohort (APC) models provides clues as to why this is, which we use to explore possible instabilities in applying Cox PH models. This model structure occurs frequently in applications such as loan credit risk, website traffic, customer churn and employee retention. Our numerical studies, designed to capture the dynamics of credit risk modeling, demonstrate that the same linear specification error from APC models occurs in Cox PH estimation when the covariates contain basis functions with linear terms, or if those covariates are correlated to the baseline hazard function. This demonstrates that the linear trend specification error in APC models is equivalent to multicollinearity instabilities in Cox PH models or in any regression context when applied to comparable problems. As part of our study of multicollinearity, we show equivalences between Cox PH estimation methods and other regression techniques, including an original proof that Breslow’s method for Cox PH estimation is equivalent to Poisson regression in the case of ties.
Copyright Infopro Digital Limited. All rights reserved.
You may share this content using our article tools. Printing this content is for the sole use of the Authorised User (named subscriber), as outlined in our terms and conditions - https://www.infopro-insight.com/terms-conditions/insight-subscriptions/
If you would like to purchase additional rights please email info@risk.net
Copyright Infopro Digital Limited. All rights reserved.
You may share this content using our article tools. Copying this content is for the sole use of the Authorised User (named subscriber), as outlined in our terms and conditions - https://www.infopro-insight.com/terms-conditions/insight-subscriptions/
If you would like to purchase additional rights please email info@risk.net