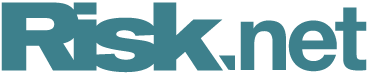
Predictive Merton
Firm-value models of default are the basis of KMV’s proprietary default prediction methodology. Recently, critics have attacked the methodology for its failure to include other potentially useful default indicators. Here, Stephen Kealhofer and Matthew Kurbat respond, arguing that such indicators fail to improve upon the KMV methodology’s predictive power.
One way to tackle this problem is through the option pricing approach to default risk, sometimes known as the Merton approach (Merton, 1974). This approach builds on the idea that an equity holder has an implicit option on the assets of a firm. The equity holder of a distressed firm can choose to limit any further liability by exercising the option to ‘put’ the assets of the firm to the debt holders.
Without going into detail, there are practical problems to be solved prior to using this approach to quantify default risk. After years of research, we and others at KMV Corporation have extended the basic Merton framework to create a practical model of default risk. The resulting model outputs a measure of Expected Default Frequency (EDF) known as the EDF Credit Measure (for details of the model, see Crosbie & Bohn, 2001).
Recently, researchers at Moody’s Investors Service have criticised the Merton approach to evaluating credit risk in several ways:
• The Merton approach is fundamentally deficient in measuring credit risk.1
•While there is credit information available in the output of a Merton model, the information can be significantly enhanced by combining it statistically with Moody’s debt ratings as well as other well-known accounting ratios.2
•The theoretical underpinnings of options pricing and thus the Merton model are incorrect.3
To back up the first two claims, Moody’s has produced statistical results showing the underperformance of a Merton approach in measuring default risk. In fact, the company has produced a new model that statistically combines its Merton approach with Moody’s ratings and accounting variables, which it shows improves the default predictive power of the Merton approach (Sobehart & Stein, 2000).
This article shows that these findings are all incorrect. In attempting to replicate Moody’s results, we find that there is no default predictive information in Moody’s ratings that is not already contained in the output of the Merton approach. Similarly, there is no additional information in the well-known accounting variables that they use. In fact, mixing Moody’s ratings and accounting variables with the output of the Merton approach does not improve its performance but rather degrades it.
Attempted replication of Moody’s empirical results
The two key empirical results that Moody’s presents are that:
• Adding Moody’s ratings and accounting variables to the output of the Merton approach significantly improves the default predictive power.
• Accounting variables and Moody’s ratings contain default predictive information, beyond the default predictive information in the Merton approach.
To evaluate these findings, we need to establish if there is any default predictive information in one measure (eg, Moody’s ratings) that is not already contained in the other measure (eg, EDF). Miller (1998) proposed an intra-cohort analysis test for this circumstance which is both intuitive and statistically powerful and does not require specifying the form of the possible relationship between the two measures. When we applied this test to a comprehensive sample of Moody’s-rated companies with public EDFs, we found that:
• All default predictive information in Moody’s ratings and accounting variables was already present in the EDFs.
• Considerable default predictive information in EDFs was not contained in Moody’s ratings or accounting variables.
•EDFs were uniformly more powerful predictors of default. In particular, they had fewer ‘false positives’ (incorrect identification of default) than the alternatives for any level of correct predictions.
The following section describes the statistical analyses performed.
Statistical methodology
Miller (1998) proposed the following statistical procedure. Take the population of firms one year prior to default and sort them into groups (‘cohorts’) according to the first measure of default risk so that, within each cohort, all firms would then have approximately the same default risk by the first measure. One year later, we will be able to see which firms actually default. If our second measure of default risk has predictive information not contained in the first measure, we should be able to find it in the following way. We sort, within each of the cohorts determined above, by the second measure. According to the first measure, each firm within a cohort should have about the same probability of defaulting. If the second measure has additional power, there should be a relatively higher default rate for the low-quality firms within each cohort, as determined by the second measure. In other words, instead of being randomly scattered within a cohort, the actual defaults should cluster among the lower credit quality firms, as determined by the second measure. Because we are looking within the cohorts formed by the first measure to find the marginal information in the second measure, this is called ‘intra-cohort analysis’.
As observed before, the intra-cohort analysis does not rely upon a particular parameterisation of the relationship between the two variables, such as a linear relationship, but rather considers all reasonable alternatives simultaneously. It simply tests for the ranking of one variable having information that is not contained in the ranking of the other variable. For this reason, it is considered a ‘non-parametric’ test. It is difficult to imagine any mathematical specification of the two variables that would be practically better than the first variable alone, if the second variable fails to have additional predictive power by this test.
Intuitively, consider grouping together all firms with EDFs of 1-2%. Interestingly, it is possible to find, in that one group, firms with agency debt ratings ranging from Aa2 to B2. However, according to the EDFs, all firms in this cohort should have approximately the same default rate. If these firms are sorted by their ratings, and if the ratings contain information not in the EDFs, then the low-rated firms in this group should default at a higher rate than the highly rated firms. If such a relationship exists, then we should be able to see it by looking at the realised default rates within each of the groups, sorted by their ratings.
To make sure that our results are meaningful and not just due to chance, we have to look at many cases. To do that, we need some way of normalising information across cohorts to be on the same scale before we combine it. We do this by converting each score within a cohort to its percentile rank, and then combining the defaults across cohorts by their percentile scores.
Table A shows how we combine results across cohorts via a hypothetical example. Assume that for each of 21 Moody’s non-defaulted rating grades, we have 10 companies, and we order the EDFs of each company from lowest (group 1) to highest (group 10). Say we also have a total of 10 defaults in the data.
If the second measure (EDFs) adds default predictive information not captured by ratings, then we would expect there to be more defaults in the higher-numbered groups than in the lower-numbered groups (eg, group 10 would have more defaults than group 1). However, if the second measure does not add default predictive information, then we would expect there to be approximately the same number of defaults in each of the groups (within limits of sampling error).
If we convert from ranks 1–10 within each cohort to percentile ranks, we can aggregate this percentile information and create histograms of percentile ranks.
Subsequently, we can apply the Kolmogorov-Smirnov (KS) statistical test to their distribution, to see if there is a statistically significant relationship due to marginal information in the second measure. The KS test works by calculating the maximum distance (D) between the observed distribution of EDFs and the uniform distribution.4 The null hypothesis is that the second measure provides no additional information, in which case the percentiles of the second measure are uniformly distributed. The alternative hypothesis is that the second measure does contain additional default predictive information, in which case we expect the percentiles of the second measure to be skewed to the right, instead of being uniformly distributed.
Results
We created a comprehensive sample of Moody’s-rated defaults from the past 10 years, where there was a rating one year before default and where the company’s equity was publicly traded one year before default. This yielded a sample of 121 rated defaults, against a population of 1,458 unique non-defaulted companies, or approximately 1,347 publicly traded, Moody’s-rated firms per year.
Using the data described above5, we formed cohorts, first using EDFs as the primary sorting variable, and then using Moody’s ratings as the primary sorting variable. That is, we first tested to see if there was marginal information in Moody’s ratings relative to EDFs, and then whether there was marginal information in EDFs relative to Moody’s ratings.
The findings are illustrated in figure 1. The left-hand graph shows that there is no pattern of default rates within the EDF cohorts due to Moody’s rating differentials. The visual lack of pattern (the histogram bars do not have a positive slope) is confirmed by the statistically insignificant KS test results. The slightly larger number of defaults on the left-hand side of the graph corresponds to firms with better-quality ratings, which is the opposite of what we would expect if ratings added predictive default power.6 In fact, out of 70 defaulting firms, 43 (more than half) have percentile ranks of 50 or below (ie, better than average credit quality ratings for the EDF cohort), which is the opposite of what we would expect if ratings added default predictive power. Firms with lower-quality Moody’s ratings within any EDF range are not more likely to default than better-rated firms in the same EDF range.
On the other hand, the right-hand graph shows that there is a very clear pattern of default rates within the Moody’s cohorts due to EDF differentials. This result is confirmed by the highly significant KS test results.7 We see that the EDF measure adds default predictive power, because the histogram bars have a positive slope. Firms with higher EDFs in any rating grade are much more likely to default than lower EDF firms in the same grade.
Therefore, our results show that there is no default predictive information in Moody’s ratings that is not already contained in EDFs, but EDFs contain substantial default predictive information that is not in ratings.
While adding EDFs to Moody’s ratings would improve ratings, adding ratings to EDFs would degrade EDFs. This result implies that the absolute default predictive power of EDFs should exceed that of Moody’s ratings.
This implication can be confirmed by performing a standard power test of EDFs versus Moody’s ratings.8 Using the same sample and one-year time horizon described above, a test of absolute default prediction power shows that EDFs are uniformly more powerful than Moody’s ratings in predicting default. These power results are shown in figure 2. A power curve shows the trade-off between type I and type II error for all possible values of the measure. The type I error is the probability of failing to identify a default in advance, and is given by the vertical distance from the chosen point to the top of the graph. The type II error is the probability of incorrectly identifying a ‘good’ firm as a default candidate, and is given by the horizontal distance to the chosen point from the origin.
The EDF power curve lies above the Moody’s rating power curve at all points, meaning that it has less type I error for any given common level of type II error, or equivalently, less type II error for any given common level of type I error. Thus EDFs are uniformly more powerful than Moody’s ratings in predicting default.
Combinations of ratings and EDFs
There is, however, a yet stronger implication of the intra-cohort analysis results. Because the intra-cohort test shows that there is no additional information in Moody’s ratings that is not already present in the EDFs, combining the two should actually reduce the default predictive power of the EDFs. Figure 3 confirms this effect of combining the two measures. The EDF alone, as implied by the intra-cohort results, outperforms the combined measure for all values of type I and type II error. The combination was created by converting both EDFs and Moody’s ratings to percentile ranks to put them on the same scale. A high EDF is a high percentile, which is a poor credit. Similarly, a rating close to ‘D’ is also a high percentile, which is a poor credit. We then simply add the two percentiles together to get the combined measure.
Return on assets as a default predictor
Moody’s has also identified the accounting variable, return on assets (ROA), as another important variable to improve the Merton model. The analysis performed above was repeated using, instead of Moody’s ratings, the accounting variable ROA. The results are summarised in figures 4 and 5. Again, there is no information in ROA that is not already contained in EDFs. Adding ROA in any fashion to EDFs only degrades the default predictive power of EDFs.
Robustness and further results
In a longer version of this paper (Kealhofer & Kurbat, 2001), we show that our results are robust across credit quality ranges, sample period9 and time horizon, and in the face of possible violations of assumptions and other limitations such as sample dependence. We also show that the same results hold for other accounting variables not presented here. Lack of space prohibits us from including those results here10, so we urge potential critics to consult the longer paper as it addresses a wide range of potential criticisms.
The results obtained here can be readily replicated by anyone using EDFs, as Moody’s ratings are publicly available.
Analysis of Moody’s empirical results
How could Moody’s have obtained results so contrary to these? One answer may be that there is not really a Merton ‘model’, but rather a Merton approach. The approach, developed by Black & Scholes (1973), Merton (1974) and others, is not a recipe for estimating credit risk, but rather a general framework. There is an infinite variety of ways in which the approach can be implemented. It is well documented in the academic literature going back 25 years that some straightforward implementations do not work very well.
The KMV model was developed over a 10-year period to address the known problems with Merton implementations. Volatility estimation is critical to obtaining good results, and KMV has developed special approaches for asset volatility estimation. These have been critical to obtaining powerful default prediction results.
Moody’s researchers are aware of these issues. For instance, Stein (2000) notes: “We use a variation on the original Merton model that has been adjusted for excess volatility. More complex versions may have different behaviour.” Nonetheless, they then proceed as if there is not different behaviour, describing their implementation as “similar [to the] adaptation of the Merton model [that] has been popularised by the KMV Corporation. For details, see Kealhofer [1991].”
What these results show is that the Merton implementation used by Moody’s does not represent the KMV implementation, and in fact has considerably less default predictive power, given how much it is improved by adding ratings and accounting variables.
Moody’s power curves suggest they have a different Merton model
What would also appear to support this interpretation are results recently published by Moody’s (Boral & Falkenstein, 2001) that compare their best Merton implementation against ROA. In contrast to our results, where EDF substantially outperforms ROA in predicting defaults, their Merton model has similar default predictive power to ROA. This again suggests that KMV’s Merton variant should substantially outperform Moody’s, although we note that these two sets of results are not perfectly comparable, because they use different samples: one uses bankruptcies and the other uses defaults, etc (see Kealhofer & Kurbat, 2001).
Too many sell signals?
There is a view (eg, Stein, 2000) that while equity prices contain information, they are also very ‘noisy’ and can thus be improved by combining them with more ‘stable’ variables such as debt ratings and accounting ratios. This criticism often takes the form that equity markets predict ‘too many’ defaults, encouraging the selling of exposures that do not subsequently default.
There are academic findings on both sides of the issue of ‘excess volatility’ in equity markets, and the debate has existed for at least the past 60 years so it will not end here. However, academics agree almost unanimously that one cannot ‘fix’ any purported excess volatility by the use of variables such as debt ratings and accounting ratios. If this was possible, then one could trade profitably with the same information.
For instance, suppose an equity price decrease indicated a higher default probability than was consistent with the debt rating, and that was interpreted as ‘noise’. One should buy that firm’s equity as the elimination of the ‘noise’ would mean a return of the equity price to a higher level. Extremely intensive testing of equity prices over the past 30 years overwhelmingly rejects the fact that variables such as those proposed by Moody’s can be used to systematically make money in equities. It is likely that equity analysts and investors have already noticed and accounted for the firm’s ROA, book leverage and so forth.
The power tests reported above confirm these results from a different perspective. Adding these variables to EDFs reduces the default predictive power of the combined measure. This means that for any given level of correct predictions of default, the combined measure will give more incorrect sell signals than EDFs alone.
Note, however, that combining a variable such as debt rating, which does not change very often, with a dynamic measure such as EDF will make the measure ‘more stable’. This will, in fact, reduce the type II error, but in an inefficient way. By ‘smoothing’ information, one is actually increasing the likelihood of failing to predict a default. One could achieve a superior result by EDFs alone, simply by setting a lower standard for rejection. This would result in the same type II error rate, but with a smaller increase in type I error rate. In other words, smoothing market price information produces inferior results.
The point is illustrated in figure 6, which ‘zooms in’ on figure 2. The type II error rate is displayed on the x-axis of the power curve graph. Point A in the chart is the 10% type II error rate point on the EDF power curve. By mixing Moody’s ratings with EDFs, the so-called ‘stabilisation’ effect is to move to a point such as B, which reduces the type II error rate. However, we have also sharply reduced correct predictions of defaults. We can obtain a superior result using EDFs alone, by simply lowering our criterion for default prediction, as in point C.
Conclusion
This article shows that the Merton approach not only uniformly outperforms Moody’s ratings and various accounting ratios in predicting default, but also appears to already contain any information in ratings or accounting ratios. Tests for marginal information from debt ratings and accounting ratios strongly indicate no additional information; tests for marginal information from the Merton approach over ratings and accounting variables are strongly positive. Clearly, KMV and Moody’s use very different variations of the Merton approach.
The Merton approach is a method for utilising information in equity prices. It is not surprising that equity prices already contain the information in accounting ratios such as ROA.
It is less obvious that equity prices should necessarily contain all the information in debt ratings. Although most bond investors believe that rating changes lag behind changes in bond prices, there are various studies that find that announcements of rating changes have an effect on stock prices. Researchers that have identified these effects (eg, Dichev & Piotroski, 2001) have not been able to show whether they are due to new fundamental information about the firm, or rather a reflection of the effect of changes in capital market access actually caused by the ratings themselves. The results here that show no marginal default predictive power of ratings relative to equity unfortunately cannot resolve this question. What they do show is that if there was new fundamental information in ratings changes, it is incorporated so rapidly into equity prices that there is no benefit from adding the rating information to the equity information.
Lastly, the results show that the Merton approach has been unfairly characterised as producing too many ‘rejections’ of firms that do not subsequently default. All the proposed alternatives to fix this ‘problem’ produce even more false rejections. Again, this is not particularly surprising because if it were not so, equity investors could earn abnormal profits by using debt ratings and ROA to guide their trades.
Stephen Kealhofer is managing director and Matthew Kurbat is a financial researcher at KMV in San Francisco. Expected Default Frequency and EDF are trademarks of KMV.
Next month Jorge Sobehart and Sean Keenan respond to Kealhofer and Kurbat’s rebuttals in the above article, reiterating their claims that pure firm-value approaches to default prediction are fundamentally flawed.
1. Stein (2000, page 1) claims that: “We provide some evidence that Merton-type models are not, in fact, complete in the sense that additional information provides better discrimination between defaulters and non-defaulters even when conditioned on Merton-based variables.” 2. For example, Sobehart & Stein (2000, page 6) claim that: “Our research has shown that Moody’s hybrid model adds predictive value over a pure continent claims approach derived from the Merton model.” See also Keenan & Sobehart 1999) and Sobehart, Keenan & Stein (2000). 3. For example, Sobehart & Keenan (1999a, page 7) claim that: “The funda- mental limitations of all the variants of the Merton model suggest the need for more general types of default risk models.” See also Sobehart & Keenan (1999b, 1999c). 4. The KS test is a basic, well-understood tool of non-parametric statistics (see, eg, Conover, 1971). Its use here is discussed further in the appendix of Keal- hofer & Kurbat (2001). 5. Power curve studies use all 121 companies mentioned above. However, sample sizes in the cohort studies are significantly smaller than 121 because, as discussed in the appendix of Kealhofer & Kurbat (2001), the cohort studies only use defaults from cohorts with at least 20 members. 6. This result appears to be due to ties in ratings, which makes the proper null distribution not exactly uniform but slightly ‘humped’ in the middle (this fact reduces even further the statistical significance of the results, as we show in the appendix of Kealhofer & Kurbat, 2001). 7. The appendix of Kealhofer & Kurbat (2001) provides more details. 8. See the appendix of Kealhofer & Kurbat (2001) for detailed description of power testing. 9. In Kealhofer & Kurbat (2001), we split the data into two sample periods containing approximately the same number of defaults. KS test results in the latter period (July 1998-December 2000) were essentially identical to those in the earlier period (January 1991-June 1998). The current version of the EDF model has been in place since the end of 1995, so the second sample period is an entirely out-of-sample test. The KS value for the second period was 0.42, slightly larger than the KS value of 0.39 presented previously for both periods combined, meaning that there is no evidence of a decline in default predictive power out of sample. 10. The original version of this paper greatly exceeds the length constraint for this journal. |
REFERENCES |
Only users who have a paid subscription or are part of a corporate subscription are able to print or copy content.
To access these options, along with all other subscription benefits, please contact info@risk.net or view our subscription options here: http://subscriptions.risk.net/subscribe
You are currently unable to print this content. Please contact info@risk.net to find out more.
You are currently unable to copy this content. Please contact info@risk.net to find out more.
Copyright Infopro Digital Limited. All rights reserved.
You may share this content using our article tools. Printing this content is for the sole use of the Authorised User (named subscriber), as outlined in our terms and conditions - https://www.infopro-insight.com/terms-conditions/insight-subscriptions/
If you would like to purchase additional rights please email info@risk.net
Copyright Infopro Digital Limited. All rights reserved.
You may share this content using our article tools. Copying this content is for the sole use of the Authorised User (named subscriber), as outlined in our terms and conditions - https://www.infopro-insight.com/terms-conditions/insight-subscriptions/
If you would like to purchase additional rights please email info@risk.net
More on Rankings
Energy Risk Commodity Rankings 2024: markets buffeted by geopolitics and economic woes
Winners of the 2024 Commodity Rankings steeled clients to navigate competing forces
Energy Risk 2024 Software Rankings: IT demands increase amid rising risk
Heightened geopolitical and credit risk increase requirements on commodities software
Hitachi Energy’s expanded portfolio steers clients through volatility
Three years after acquiring US-based commodities trading and risk management software firm Pioneer Solutions, Hitachi Energy is reaping the rewards of its large portfolio
Ensuring energy technology keeps up with the pace of change
Change may well be a constant in energy markets, but its velocity over the past 18 months has been extraordinary. This has seen KWA Analytics’ clients race to ensure their ETRM infrastructure can keep up – and be ready for change yet to come. Directors…
UBS precious metals team shines amid market turmoil
Global uncertainty always adds allure to precious metals, putting a premium on the long-standing relationships and cutting-edge technology of the UBS precious metals team
Enabling green choices despite market turmoil
Throughout recent market tumult, ENGIE remained committed to net zero, forging even closer connections with clients to fuel global growth
Energy Risk Commodity Rankings 2023: Axpo – the power of partnerships
In the face of recent extreme volatility and price hikes, the strong partnerships Axpo has with its clients has enabled both parties to weather the storm
ION Commodities puts customer engagement at the heart of its business
Sponsored content
Most read
- Breaking out of the cells: banks’ long goodbye to spreadsheets
- Too soon to say good riddance to banks’ public enemy number one
- Industry calls for major rethink of Basel III rules