Journal of Risk Model Validation
ISSN:
1753-9579 (print)
1753-9587 (online)
Editor-in-chief: Steve Satchell
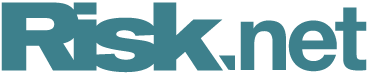
Default prediction based on a locally weighted dynamic ensemble model for imbalanced data
Need to know
- An accurate prediction model is required for financial risk management.
- The locally weighted dynamic ensemble model (LWDE) model improves the diversity and generalisability of balanced bagging.
- Our LWDE model incorporates a new ensemble learning strategy.
- The proposed model outperforms the other baseline models, and can predict five years.
Abstract
Default prediction plays a decisive role in the credit decisions of financial institutions. To avoid the bias that can occur in model predictions due to differences in the numbers of defaulting and nondefaulting firms, this study proposes a locally weighted dynamic ensemble model. To construct more diverse base classifiers, ten imbalanced data sampling methods and five heterogeneous classifiers are introduced to balanced bagging to select the base classifiers with the highest accuracy under different data distributions. To reduce overfitting and information loss, the locally weighted dynamic ensemble method is used to obtain the final prediction result. Experiments on three publicly available data sets and a data set of Chinese listed firms validate that the predictive performance of the proposed ensemble model is superior to that of three other heterogeneous ensemble models, seven homogeneous ensemble models and five individual models in predicting the imbalanced data. Moreover, the proposed ensemble model can predict financial institutions’ default status five years ahead.
Copyright Infopro Digital Limited. All rights reserved.
You may share this content using our article tools. Printing this content is for the sole use of the Authorised User (named subscriber), as outlined in our terms and conditions - https://www.infopro-insight.com/terms-conditions/insight-subscriptions/
If you would like to purchase additional rights please email info@risk.net
Copyright Infopro Digital Limited. All rights reserved.
You may share this content using our article tools. Copying this content is for the sole use of the Authorised User (named subscriber), as outlined in our terms and conditions - https://www.infopro-insight.com/terms-conditions/insight-subscriptions/
If you would like to purchase additional rights please email info@risk.net