Journal of Risk Model Validation
ISSN:
1753-9579 (print)
1753-9587 (online)
Editor-in-chief: Steve Satchell
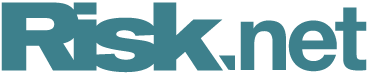
Comprehensive Capital Analysis and Review consistent yield curve stress testing: from Nelson–Siegel to machine learning
Need to know
- In stress testing, especially when using a top-down approach, it is common to design scenarios for a core set of variables. When applying these scenarios to a specific area, one needs to translate the changes in the core variables to additional variables that better capture specific risk. In this paper, we clearly define a scenario translation problem for interest rate variables. The same idea can be applied to other situations when one needs to expand a list of stressed variables.
- Given the need to estimate additional variables, considerable effort is given to determining the most efficient approach incorporating both computational time and accuracy of results. By examining three different methods one can choose the approach that best suits their needs. Our analysis results in three viable alternatives to solving the translation problem for interest rate variables.
- ANN did not significantly outperform PCA, with its performance likely constrained by dataset size. PCA draws a good balance between complexity and performance, compared to the other two approaches. Outside of CCAR, ANN is promising when simulated data is utilized and inherent factors in the dataset become more complex. Its flexibility and ability to generalize makes it a good candidate for generic stress testing problems.
Abstract
Following the global financial crisis of 2007–9, the regulators established a stress testing framework known as Comprehensive Capital Analysis and Review (CCAR). The regulatory stress scenarios in this framework are macroeconomic and do not define stress values for all the relevant risk factors. In particular, only three Treasury rates are captured in these scenarios. CCAR scenarios can be complemented by defining stress values for the missing risk factors. The Treasury rates corresponding to different nodes are highly correlated. Hence, the changes in the three Treasury rates defined in the regulatory scenarios may impact the other rates. This paper focuses on CCAR-consistent Treasury yield curve stress testing. We assessed via backtesting three modeling approaches that allow us to “build” the stressed curves under CCAR scenarios: the Nelson–Siegel approach, principal component analysis (PCA) and the artificial neural network approach. The PCA approach fits the scenario-generation problem better than Nelson–Siegel because it explicitly takes into consideration correlation among historical changes in rates corresponding to different nodes, while the artificial neural network approach allows us to directly link the changes in the three Treasury rates to the changes in the other rates.
Copyright Infopro Digital Limited. All rights reserved.
You may share this content using our article tools. Printing this content is for the sole use of the Authorised User (named subscriber), as outlined in our terms and conditions - https://www.infopro-insight.com/terms-conditions/insight-subscriptions/
If you would like to purchase additional rights please email info@risk.net
Copyright Infopro Digital Limited. All rights reserved.
You may share this content using our article tools. Copying this content is for the sole use of the Authorised User (named subscriber), as outlined in our terms and conditions - https://www.infopro-insight.com/terms-conditions/insight-subscriptions/
If you would like to purchase additional rights please email info@risk.net