Journal of Computational Finance
ISSN:
1460-1559 (print)
1755-2850 (online)
Editor-in-chief: Christoph Reisinger
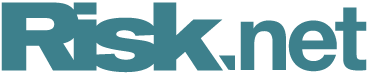
Finding the nearest covariance matrix: the foreign exchange market case
Need to know
- We put forward a simple algorithm for rescaling the optimisation problem of finding a nearest correction to an empirical covariance matrix of asset returns
- The reduction technique applies only to the case of an FX market, where the common no-arbitrage conditions in currency triangles must be taken into account
- With the size of the problem reduced one can apply a greater variety of numerical methods to find its solution
- We compare performance of several such methods and demonstrate an average increase in speed of up to 500 times in resolving problems of medium scale
Abstract
We consider the problem of finding a valid covariance matrix in the foreign exchange market given an initial nonpositively semidefinite (non-PSD) estimate of such a matrix. The common no-arbitrage assumption imposes additional linear constraints on such matrixes, inevitably making them singular. As a result, even the most advanced numerical techniques will predictably balk at a seemingly standard optimization task. The reason is that the problem is ill posed, while its PSD solution is not strictly feasible. In order to deal with this issue we describe a low-dimensional face of the PSD cone that contains the feasible set. After projecting the initial problem onto this face, we come out with a reduced problem, which is both well posed and of a smaller scale. We show that, after solving the reduced problem, the solution to the initial problem can be recovered uniquely in one step. We run numerous numerical experiments to compare the performance of different algorithms in solving the reduced problem and to demonstrate the advantages of dealing with the reduced problem as opposed to the original one. The smaller scale of the reduced problem implies that its solution can effectively be found by the application of virtually any numerical method.
Copyright Infopro Digital Limited. All rights reserved.
You may share this content using our article tools. Printing this content is for the sole use of the Authorised User (named subscriber), as outlined in our terms and conditions - https://www.infopro-insight.com/terms-conditions/insight-subscriptions/
If you would like to purchase additional rights please email info@risk.net
Copyright Infopro Digital Limited. All rights reserved.
You may share this content using our article tools. Copying this content is for the sole use of the Authorised User (named subscriber), as outlined in our terms and conditions - https://www.infopro-insight.com/terms-conditions/insight-subscriptions/
If you would like to purchase additional rights please email info@risk.net