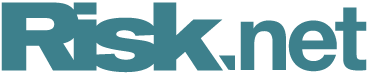
Skew this: taking the computational burden off basket options
Dan Pirjol presents a snap formula for estimating implied volatility skew in an instant
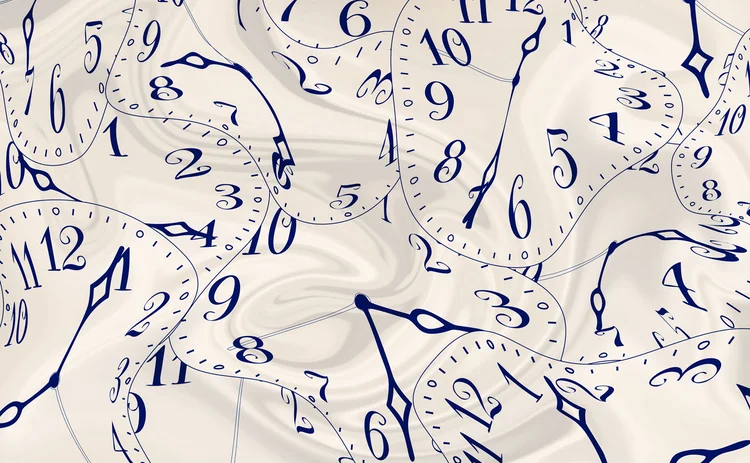
The consensus on basket options is that they are both useful and a headache.
Useful because buying an option on a basket is cheaper than hedging the constituents individually. The headache is estimating the skew or slope of the implied volatility smile, which for basket options depends on the volatilities and correlations of the underlying assets. This is computed by running Monte Carlo simulations – a laborious process that effectively limits the number of underlyings and rules out dynamic trading.
That could be about to change. Dan Pirjol, associate professor at the Stevens Institute of Technology, has developed a closed-form formula that estimates the volatility skew of a basket option almost instantaneously, regardless of the number of constituents.
His paper, Smile-consistent basket skew, could make it possible to quickly create options on larger baskets and to compare the skew of baskets and components to determine if either is overpriced.
“This paper retrieves a formula that is quite simple to use and [provides] an approximation of the ATM skew for the basket options,” says Valer Zetocha, senior quant for equity and FX at Julius Baer.
He thinks it could be useful for dealing with large numbers of baskets, or baskets that are large in size – or both. “For example, when building a basket that is optimised for a given metric, this formula allows for a very quick evaluation of the skew of the resulting basket of each combination of components,” he says. “It can also be used to estimate the difference between the implied skew of a large index and that implied by index constituents.”
Pirjol’s paper is based on the classic local volatility model and builds on the previous work of Marco Avellaneda and others. “They found a formula for pricing basket options at short maturities and/or volatilities,” says Pirjol. The problem with that approach is that it requires a numerical solution – the optimisation problem must be solved for each valuation, which for large baskets can become computationally prohibitive.
Still, Pirjol considers the model developed by Avellaneda and his co-authors to be the gold standard for pricing basket options. Other approaches such as the copula or moment matching are more ad hoc and not guaranteed to produce the correct result in the small maturity or volatility limit.
“I simplified Avellaneda’s approach,” he says. “Rather than computing the entire volatility smile of a basket, the method provides only the at-the-money skew. For that, you can solve the optimisation problem analytically and write down a closed-form formula for the skew. For a long time, we had a simple formula for the at-the-money volatility for basket options that is widely used in practice. Now I extend this to the at-the-money skew.”
The formula is remarkably simple. The only required inputs are the weights of the basket components, their ATM vols and skews and the correlation matrix. No stochastic calculus or simulations are involved and, crucially, the entire smile for each component is not required, resulting in speedier calculations.
Rather than computing the entire volatility smile of a basket, the method provides only the at-the-money skew
Dan Pirjol, Stevens Institute of Technology
“When applied to index options, it gives the index skew in terms of the vols and skews of the index components. It goes into the granular composition of the index. If the index components are known, the model can estimate the index skew,” says Pirjol.
The results so far are promising: the paper shows that for positive correlations, the margin of error is comparable or smaller than for Monte Carlo simulations. The examples in the paper deal with simple baskets with two or three constituents, but Pirjol says his tests on instruments with 20 components returned similar results. He believes the model is numerically accurate for any number of underlyings.
Pirjol agrees with Zetocha that the model can be used to estimate the correlation risk premium, which explains why the skew of baskets tends to be underpriced relative to its components. “Intuitively, the discrepancy is due to the market participants' expectations of the future correlation,” he says. “The approach proposed here could help estimate this premium.”
He also thinks the model can provide valuable information to buy-side firms that want to assess how much of the observed skew of a portfolio is due to its components versus the correlation expectations of market participants.
Only users who have a paid subscription or are part of a corporate subscription are able to print or copy content.
To access these options, along with all other subscription benefits, please contact info@risk.net or view our subscription options here: http://subscriptions.risk.net/subscribe
You are currently unable to print this content. Please contact info@risk.net to find out more.
You are currently unable to copy this content. Please contact info@risk.net to find out more.
Copyright Infopro Digital Limited. All rights reserved.
You may share this content using our article tools. Printing this content is for the sole use of the Authorised User (named subscriber), as outlined in our terms and conditions - https://www.infopro-insight.com/terms-conditions/insight-subscriptions/
If you would like to purchase additional rights please email info@risk.net
Copyright Infopro Digital Limited. All rights reserved.
You may share this content using our article tools. Copying this content is for the sole use of the Authorised User (named subscriber), as outlined in our terms and conditions - https://www.infopro-insight.com/terms-conditions/insight-subscriptions/
If you would like to purchase additional rights please email info@risk.net
More on Views
Filling gaps in market data with optimal transport
Julius Baer quant proposes novel way to generate accurate prices for illiquid maturities
Georgios Skoufis on RFRs, convexity adjustments and Sabr
Bloomberg quant discusses his new approach for calculating convexity adjustments for RFR swaps
A dynamic margin model takes shape
New paper shows how creditworthiness and concentrations can be reflected into margin requirements
How HSBC got better at pricing share buy-backs
Monte Carlo approach generates faster, more reliable pricing for complex deals
Degree of influence 2023: Quants thrive on volatility
Climate, crypto and market impact also featured among the top research topics in 2023
How to account for banks’ contribution to CO2 emissions
Price adjustments will depend on individual counterparties’ carbon footprints
Exploiting causal biases in market impact models
Model calibration gains efficiency by including biased but adjusted trading data
How a machine learning model closed a hidden FX arbitrage gap
MUFG Securities quant uses variational inference to control the mid volatility of options