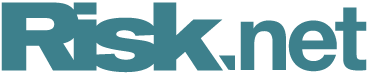
Reviving the lost art of perturbation for exotic pricing
Natixis quants find novel way to speed up volatility smile modelling
Banks must perform a delicate balancing act when pricing exotic products. They must respond quickly to quote requests from clients – a delay of five minutes might be enough to lose a deal – and they need to be accurate. Quoting too high a price might lead to no deal, going too low might create arbitrage opportunities and a subsequent loss.
To price accurately, banks need to model the impact of the dynamics of the volatility smile on the book P&L. Local stochastic volatility (LSV) models are the standard tool for this, but they are computationally intensive, which limits speed. Even so, LSV is “a necessity” when pricing exotics, “because it describes the cost of both static and dynamic hedging of the smile”, says Adil Reghai, head of the quantitative research team for equities, commodities and hybrid business at Natixis.
To balance speed with accuracy, banks can either cut computation corners, deploy additional hardware or – as many have done more recently – adopt deep-learning approaches to hasten some steps in the process.
Reghai and a quantitative analyst in his team, Florian Monciaud, have developed an alternative model built on local volatility (LV), Greeks and perturbation techniques that is faster than LSV while producing comparable results.
The key variable in their method is the difference between the LSV and the LV price. By modelling it, they enable the approximation of the LSV price by addition of the LV price (which is computationally less intensive to obtain than the LSV price) and the LSV-LV component.
LV models work well for vanilla products pricing, but are unsuitable for capturing the smile dynamics, which is necessary for exotic path-dependent products such as autocallables. Reghai and Monciaud hit upon the idea of starting with the LV model to build the LSV model.
That can be done by perturbing the parameters of the model to assess the impact of the smile dynamics on the P&L. In the case of LV and LSV, it turns out that a standard perturbation approach would cause undesirable effects when pricing vanilla products. Because LV is already a good pricer for vanilla products, additional terms that are supposed to be non-zero are likely to affect the calibration. Reghai and Monciaud found a novel solution to the problem.
“This is where we found out that exotic Greeks is the right concept for solving that issue. An exotic Greek is a Greek that is associated with the vanilla hedged position. It is non-zero for path-dependent options and exactly zero for vanilla options,” Reghai explains.
This exotic Greek can be obtained by exploiting a “well-chosen” scenario, which essentially means calibrating the LV model to a special implied volatility surface designed to capture the exotic-ness of the product.
The result is a P&L that is a function of the LV price and exotic Greeks of the second order gamma, vanna and volga.
“The classic local approach doesn’t guarantee to match the vanilla exactly. There can always be an error that you can only cancel at a very high computational cost. Whereas, this method, because it’s based on local vol prices, converges very well,” Reghai explains.
The computational advantage over the standard LSV model becomes apparent once the calibration time is taken into account. “The job is to imply the stochastic volatility parameters in LSV for autocalls. It was taking hours for each underlying, because you have to optimise a non-convex problem. Now, it’s taking a few minutes for all the set of around a thousand underlyings,” says Reghai.
The lost art
The approach is winning positive early reviews from quants.
“Add-on methods like this are well-known on trading desks. Adil formalised this idea, generalised it and put it on a solid mathematical ground. Traders like this type of approaches because computationally it’s faster and more stable. And they like that it’s explainable,” says Antoine Savine, chief quantitative analyst at Danske Bank.
“Perturbation techniques are a lost art,” says Vladimir Lucic, visiting professor at Imperial College London and experienced volatility quant. “It’s good to see them coming back for important applications.” However, he questions whether the speed boost comes at the cost of accuracy in stressed markets.
“I feel that is a relevant question, especially now that compute power has become more affordable, and there have been significant advances in machine learning approaches designed to drastically reduce it,” he says.
Reghai says any slippage is minimal. “The difference is one or two basis points, which is very small compared to an impact that we’re trying to capture, which is around 200bp. We can say that we get 99% of the official impact.”
The model is already used at Natixis to produce quotations for exotics such as autocallables, with plans to extend it to other products: “Future work is about applying the same technology for multi assets, because while we have a great proportion of deals on single underlyings, there is a non-negligible portion that is written on three, five or sometimes up to 50 underlyings.”
Only users who have a paid subscription or are part of a corporate subscription are able to print or copy content.
To access these options, along with all other subscription benefits, please contact info@risk.net or view our subscription options here: http://subscriptions.risk.net/subscribe
You are currently unable to print this content. Please contact info@risk.net to find out more.
You are currently unable to copy this content. Please contact info@risk.net to find out more.
Copyright Infopro Digital Limited. All rights reserved.
You may share this content using our article tools. Printing this content is for the sole use of the Authorised User (named subscriber), as outlined in our terms and conditions - https://www.infopro-insight.com/terms-conditions/insight-subscriptions/
If you would like to purchase additional rights please email info@risk.net
Copyright Infopro Digital Limited. All rights reserved.
You may share this content using our article tools. Copying this content is for the sole use of the Authorised User (named subscriber), as outlined in our terms and conditions - https://www.infopro-insight.com/terms-conditions/insight-subscriptions/
If you would like to purchase additional rights please email info@risk.net
More on Views
Podcast: Olivier Daviaud on P&L attribution for options
JP Morgan quant discusses his alternative to Greeks decomposition
Filling gaps in market data with optimal transport
Julius Baer quant proposes novel way to generate accurate prices for illiquid maturities
Georgios Skoufis on RFRs, convexity adjustments and Sabr
Bloomberg quant discusses his new approach for calculating convexity adjustments for RFR swaps
A dynamic margin model takes shape
New paper shows how creditworthiness and concentrations can be reflected into margin requirements
How HSBC got better at pricing share buy-backs
Monte Carlo approach generates faster, more reliable pricing for complex deals
Degree of influence 2023: Quants thrive on volatility
Climate, crypto and market impact also featured among the top research topics in 2023
How to account for banks’ contribution to CO2 emissions
Price adjustments will depend on individual counterparties’ carbon footprints
Exploiting causal biases in market impact models
Model calibration gains efficiency by including biased but adjusted trading data
Most read
- Podcast: Olivier Daviaud on P&L attribution for options
- For a growing number of banks, synthetics are the real deal
- SG trader dismissals shine spotlight on intraday limit controls