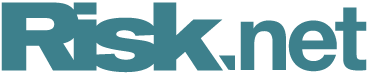
Machine learning, post-Brexit novation and a world after Libor
The week on Risk.net, March 10-16 2018

Quants warn over flaws in machine-learning predictions
Six quants debate whether the tool can adjust to paradigm shifts in financial markets
Brexit: banks take the ‘no’ out of novations
Swaps clauses stop end-users blocking counterparty switch, making it easier to move trades to EU affiliates
After Libor: Japan, Australia look to multi-rate future
Using new risk-free rates alongside Libor equivalents gains industry support
COMMENTARY: Don’t rage against the machine
As the machine-learning boom continues, several quants have begun to warn of its flaws and hazards. They are not wrong to do so. As they point out, the training data set is limited (there’s only one set of historical market prices), and basing forecasts entirely on past data leaves you vulnerable to a sudden shift in market behaviour.
In a time when machine learning is being leant on to resolve issues such as model risk, as well as provide new insights into short-term stock price changes, it is of course worth delivering an occasional note of caution.
But it’s also worth asking: what makes us think humans can do any better? Humans also have only one set of data on historical market prices to learn from, and tend to privilege recent experience, or, worse, data that backs up their existing assumptions. And if critics emphasise the value of human intuition over machine learning, consider this: what have those intuition-based assumptions done for us lately?
The stock price study in question found that one widely held assumption – the belief that intraday pricing and risk models should be sector-specific – was simply not supported by the data. There is an entire subgenre of literature (with Daniel Kahneman’s excellent Thinking, Fast and Slow at its head) that lists and analyses the countless ways in which human intuition can be led astray.
The kind of casual, rapid heuristics that served humans well for most of history simply aren’t fit to handle the massive and complex data landscapes of modern financial markets unaided. In the financial crisis, 10 years ago we saw a very good example of this. Yes, machines are not good at dealing with sudden step changes in market dynamics – but neither are people. Machines, at least, are steadily getting better at it.
STAT OF THE WEEK
The biggest operational risk loss in February was incurred by US insurer MetLife, which reinstated $510 million of pension reserves it had previously released in the 1990s. The firm discovered it had failed to make appropriate efforts to locate almost 13,500 people before declaring them “unresponsive and missing” and releasing their funds from its reserves.
QUOTE OF THE WEEK
“There is a natural bias toward optimism. Most firms that are in the doldrums will have a route map out of that and will convince themselves and their auditors that a downward trend isn’t going to continue. It would be surprising if someone said ‘my view of the future is calamitous, and therefore I’m going to voluntarily have significantly higher provisions than the neutral projections would indicate’” – Adrian Docherty, BNP Paribas
Further reading
Only users who have a paid subscription or are part of a corporate subscription are able to print or copy content.
To access these options, along with all other subscription benefits, please contact info@risk.net or view our subscription options here: http://subscriptions.risk.net/subscribe
You are currently unable to print this content. Please contact info@risk.net to find out more.
You are currently unable to copy this content. Please contact info@risk.net to find out more.
Copyright Infopro Digital Limited. All rights reserved.
You may share this content using our article tools. Printing this content is for the sole use of the Authorised User (named subscriber), as outlined in our terms and conditions - https://www.infopro-insight.com/terms-conditions/insight-subscriptions/
If you would like to purchase additional rights please email info@risk.net
Copyright Infopro Digital Limited. All rights reserved.
You may share this content using our article tools. Copying this content is for the sole use of the Authorised User (named subscriber), as outlined in our terms and conditions - https://www.infopro-insight.com/terms-conditions/insight-subscriptions/
If you would like to purchase additional rights please email info@risk.net
More on 7 days in 60 seconds
Bank capital, margining and the return of FX
The week on Risk.net, December 12–18
Hedge fund losses, CLS and a capital floor
The week on Risk.net, December 5–11
Capital buffers, contingent hedges and USD Libor
The week on Risk.net, November 28–December 4
SA-CCR, SOFR lending and model approval
The week on Risk.net, November 21-27, 2020
Fallbacks, Libor and the cultural risks of lockdown
The week on Risk.net, November 14-20, 2020
Climate risk, fixing Libor and tough times for US G-Sibs
The week on Risk.net, November 7-13, 2020
FVA pain, ethical hedging and a degraded copy of Trace
The week on Risk.net, October 31–November 6, 2020
Basis traders, prime brokers and election risk
The week on Risk.net, October 24-30, 2020
Most read
- Breaking out of the cells: banks’ long goodbye to spreadsheets
- Too soon to say good riddance to banks’ public enemy number one
- Industry calls for major rethink of Basel III rules